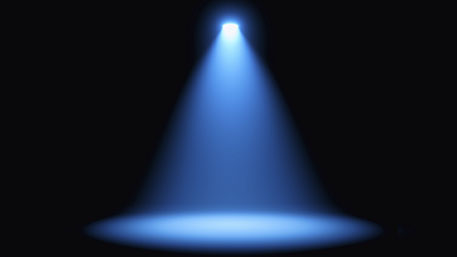
Development and Validation of an 18-Gene Urine Test for High-Grade Prostate Cancer
Genomic risk scores in prostate cancer: polygenic yes, but are they poly-ancestral?
Arnab Basu et al. J Natl Cancer Inst 2024 2
Forget lung, breast or prostate cancer: why tumour naming needs to change The conventional way of classifying metastatic cancers according to their organ of origin is denying people access to drugs that could help them.
F Andre et al, Nature, January 31, 2024
Genetic risk and likelihood of prostate cancer detection on first biopsy by ancestry.
Kyung Min Lee et al. J Natl Cancer Inst 2024 1
Disclaimer: Articles listed in the Public Health Genomics and Precision Health Knowledge Base are selected by the CDC Office of Public Health Genomics to provide current awareness of the literature and news. Inclusion in the update does not necessarily represent the views of the Centers for Disease Control and Prevention nor does it imply endorsement of the article's methods or findings. CDC and DHHS assume no responsibility for the factual accuracy of the items presented. The selection, omission, or content of items does not imply any endorsement or other position taken by CDC or DHHS. Opinion, findings and conclusions expressed by the original authors of items included in the update, or persons quoted therein, are strictly their own and are in no way meant to represent the opinion or views of CDC or DHHS. References to publications, news sources, and non-CDC Websites are provided solely for informational purposes and do not imply endorsement by CDC or DHHS.
- Page last reviewed:Feb 1, 2024
- Page last updated:Apr 25, 2024
- Content source: