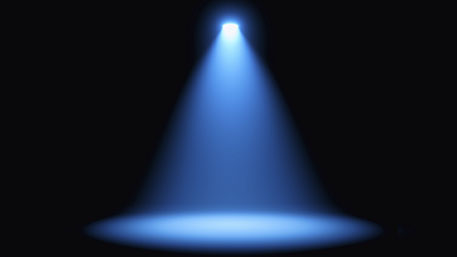
Early dementia diagnosis: blood proteins reveal at-risk people The results of a large-scale screening study could be used to develop blood tests to diagnose diseases such as Alzheimer’s before symptoms take hold.
M Naddaf, Nature, February 13, 2024
Assessment of Mendelian and risk factor genes in Alzheimer disease: a prospective nationwide clinical utility study and recommendations for genetic screening
G Nicolas et al, Genetics in Med, January 24, 2024
Direct to Consumer Biomarker Testing for Alzheimer Disease—Are We Ready for the Insurance Consequences?
JJ Arias et al, JAMA Neurology, December 18, 2023
Attitudes toward pre-symptomatic screening for Alzheimer’s dementia in five European countries: a comparison of family members of people with Alzheimer’s dementia versus non-family members
IA Angelidou et al, Frontiers Genetics, December 2023
Disclaimer: Articles listed in the Public Health Genomics and Precision Health Knowledge Base are selected by the CDC Office of Public Health Genomics to provide current awareness of the literature and news. Inclusion in the update does not necessarily represent the views of the Centers for Disease Control and Prevention nor does it imply endorsement of the article's methods or findings. CDC and DHHS assume no responsibility for the factual accuracy of the items presented. The selection, omission, or content of items does not imply any endorsement or other position taken by CDC or DHHS. Opinion, findings and conclusions expressed by the original authors of items included in the update, or persons quoted therein, are strictly their own and are in no way meant to represent the opinion or views of CDC or DHHS. References to publications, news sources, and non-CDC Websites are provided solely for informational purposes and do not imply endorsement by CDC or DHHS.
- Page last reviewed:Feb 1, 2024
- Page last updated:Apr 25, 2024
- Content source: