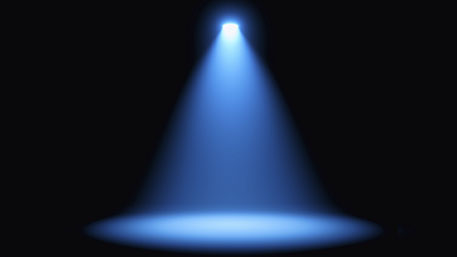
Early adverse physiological event detection using commercial wearables: challenges and opportunities
Utilizing geospatial artificial intelligence to map cancer disparities across health regions
A Fadiel et al, Sci Report, April 2, 2024
Deep learning in cancer genomics and histopathology
M Unger et al, Genome Medicine, March 27, 2024
Disclaimer: Articles listed in the Public Health Genomics and Precision Health Knowledge Base are selected by the CDC Office of Public Health Genomics to provide current awareness of the literature and news. Inclusion in the update does not necessarily represent the views of the Centers for Disease Control and Prevention nor does it imply endorsement of the article's methods or findings. CDC and DHHS assume no responsibility for the factual accuracy of the items presented. The selection, omission, or content of items does not imply any endorsement or other position taken by CDC or DHHS. Opinion, findings and conclusions expressed by the original authors of items included in the update, or persons quoted therein, are strictly their own and are in no way meant to represent the opinion or views of CDC or DHHS. References to publications, news sources, and non-CDC Websites are provided solely for informational purposes and do not imply endorsement by CDC or DHHS.
- Page last reviewed:Feb 1, 2024
- Content source: