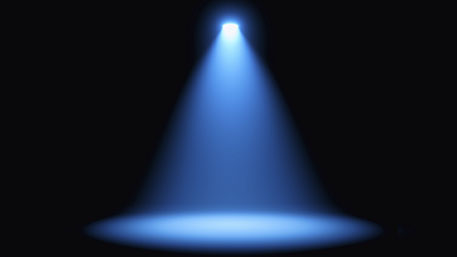
Vaccine effectiveness against emerging COVID-19 variants using digital health data
International perspectives on measuring national digital public health system maturity through a multidisciplinary Delphi study
L Ma et al, NPJ Digital Medicine, April 12, 2024
Improved measurement of disease progression in people living with early Parkinson's disease using digital health technologies.
Matthew D Czech et al. Commun Med (Lond) 2024 3 (1) 49
Disclaimer: Articles listed in the Public Health Genomics and Precision Health Knowledge Base are selected by the CDC Office of Public Health Genomics to provide current awareness of the literature and news. Inclusion in the update does not necessarily represent the views of the Centers for Disease Control and Prevention nor does it imply endorsement of the article's methods or findings. CDC and DHHS assume no responsibility for the factual accuracy of the items presented. The selection, omission, or content of items does not imply any endorsement or other position taken by CDC or DHHS. Opinion, findings and conclusions expressed by the original authors of items included in the update, or persons quoted therein, are strictly their own and are in no way meant to represent the opinion or views of CDC or DHHS. References to publications, news sources, and non-CDC Websites are provided solely for informational purposes and do not imply endorsement by CDC or DHHS.
- Page last reviewed:Feb 1, 2024
- Content source: